
The realities of GenAI in Software Development
Introduction: the promise and pitfalls of GenAI for developers
Coding with Generative AI (GenAI) systems is quickly becoming a trend. We frequently hear about the benefits of coding with GenAI, often supported by claims of productivity boosts. While the exact figures vary, estimates range from a 10–30% increase today to as much as 45% over the next five years.
Beyond these forecasts, the reality is that GenAI is already making a tangible impact. For example, Amazon reports that Java upgrades are no longer a concern, as they can now be fully automated. Mark Zuckerberg has announced plans to replace Meta’s mid-level engineers with AI this year, and Sam Altman predicts that the GenAI revolution in software development will be well underway by the end of 2025.
There are many more examples—just do a quick Google search or ask ChatGPT for sources, and you’ll find dozens, if not hundreds, of similar stories.
While using GenAI for coding offers clear advantages, it also presents challenges. In this article, we’ll share our experience at IriusRisk—what worked, what didn’t, and what we learned along the way.
The top three risks of using GenAI for Code creation
As technology leaders, CTOs are responsible for driving the adoption of technologies that can improve how a company operates—but without falling into traps. It’s essential to balance short-, mid-, and long-term impact, carefully considering the trade-offs involved.
Using GenAI for coding brings clear benefits, but it also comes with some serious challenges. From our experience, these challenges fall into three main categories:
- Bugs and Hallucinations:
- The generated code may look fine but be riddled with bugs—or it might not even compile.
- Sometimes, you’ll find surprising things like API calls, functions, or entire libraries that simply don’t exist.
- Security Vulnerabilities:
- GenAI can easily produce code that introduces security flaws.
- Most models aren’t trained with secure coding practices in mind (e.g., OWASP, NIST).
- Security-critical decisions often need to be spelled out explicitly. For example: “I’m storing sensitive data in an S3 bucket—it must be encrypted.”
- Loss of understanding and clarity by the developer:
- When GenAI generates large chunks of code, developers can lose track of what’s really going on. The result? Code that might work, but is unstable, inefficient, hard to maintain or full of hidden bugs.
Thinking about these It always come to my mind the next scene of Will Smith’s I Robot movie:
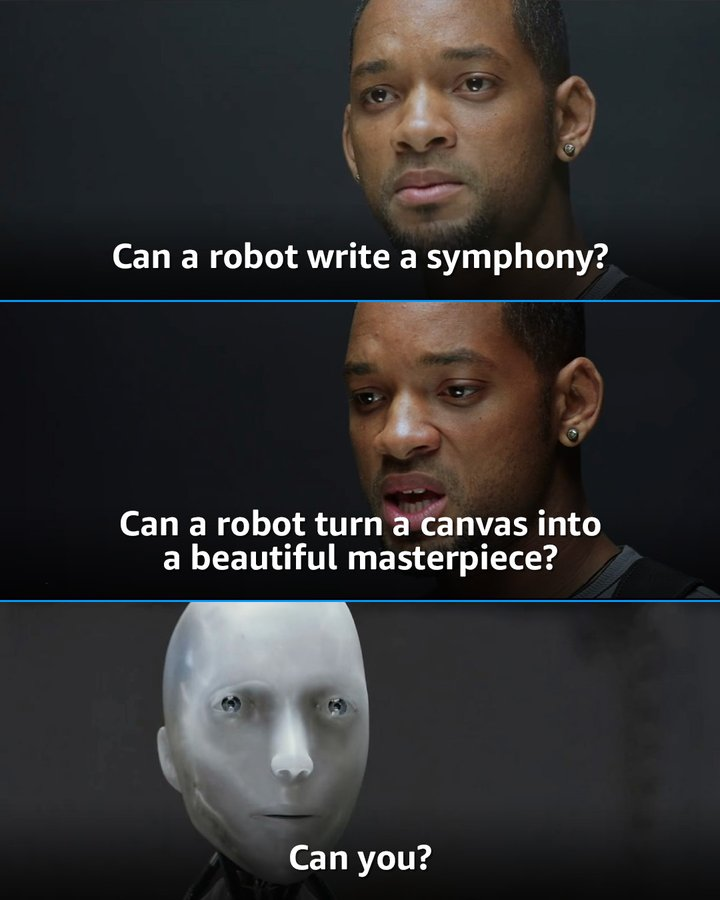
And let’s be honest—if a developer isn’t experienced enough, they can fall for the same traps GenAI does.
So, what really matters here? What has always set apart a good developer from a not-so-good one?
You got it—skills and process.
How IriusRisk uses GenAI: process, lessons, and results
Some time ago, I took a course by Jeremy Howard (fast.ai), and it really stuck with me. I learned how to use GenAI for coding in an iterative way—maintaining context, staying in control, and still getting that productivity boost. That’s when it clicked: the process is the key.
When we talk about “GenAI coding,” it’s not about throwing a vague prompt at the model like:
"I want to develop an e-commerce app that lets users register and buy products I upload through a backend."
That’s not how it works—not if you want quality.
The right approach looks very different:
A well-crafted, iterative process. One where you stay in control, set boundaries, and guide the AI through small, purposeful steps—backed by constant validation and rigorous testing. That’s where the magic happens.
At IriusRisk, we’re using GenAI for coding—but it’s been a learning journey that’s helped us uncover where it really makes a difference for us.
Recently, we completed a major backend refactor involving over a million lines of code. We approached it with an AI-assisted workflow: feeding in a class or a set of classes, applying a predefined series of prompts, testing and validating the output, and then merging it into our codebase.
The result? Based on our internal metrics, we saw a 40–60% productivity boost. Or, put another way—we finished the refactor in half the time we originally estimated.
We’ve also seen great results in smaller, focused projects. In one case, we used Cursor combined with Claude Sonnet 3.7 MAX to refactor an entire small backend in under a week—a task we had forecasted to take about a month. And importantly, the developer remained fully in control, with a clear understanding of everything happening under the hood.
That said, we’ve also learned where GenAI shines—and where it doesn’t. These productivity gains hold up when the task is structured, repetitive, and scoped—or when you're working with existing well-structured code. But for new features or greenfield development, things get trickier. In those cases, we’ve seen only a 10–15% productivity increase—and not without risks.
Summary: coordinate a company-wide framework
GenAI has proven genuinely helpful for us—especially with scoped, repetitive tasks like controlled refactoring. It works best when the codebase is well-structured and clean, allowing the model to follow patterns reliably.
It's also useful for rapid prototyping, as long as you're aware that these prototypes are essentially 100% technical debt.
To truly benefit from GenAI in coding, you need a company-wide framework. Without one, it's hard to keep your codebase clean, manageable, and ready to evolve at speed.
We believe we're still a long way from autonomous GenAI coding replacing mid-level—let alone junior—developers. But if Alan Turing were still around, even he would be like, ‘Wait, it can do that?’

FAQs
What are the top three risks of using GenAI for Code creation?
The top three risks when using GenAI for code creation are bugs and hallucinations:, security vulnerabilities, and loss of understanding and clarity by the developer.
Can you use GenAI safely in software development?
Yes, you can use GenAI safely in software development—especially for scoped, repetitive tasks and rapid prototyping. BUT only if you have a strong company-wide framework to keep your codebase clean, structured, and manageable.
Will Gen AI replace software developers?
No, GenAI won’t replace software developers anytime soon—it can significantly boost productivity for structured, repetitive tasks, but real coding still demands skilled developers who guide, validate, and manage the process carefully to avoid bugs, security risks, and loss of code clarity.